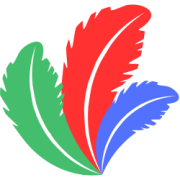
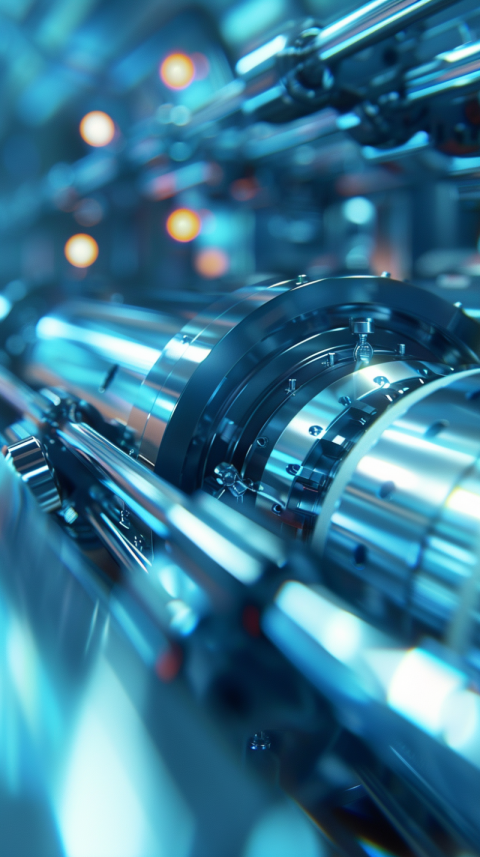
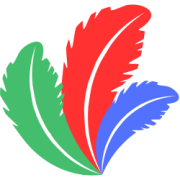
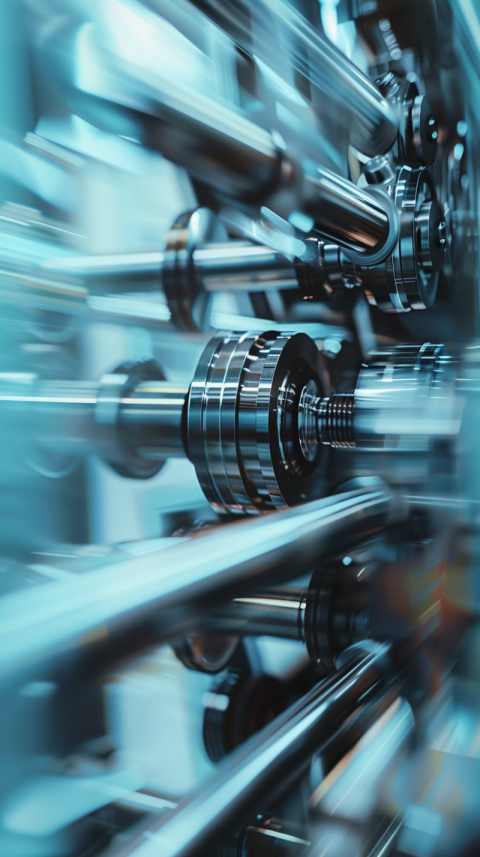
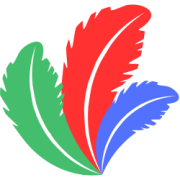
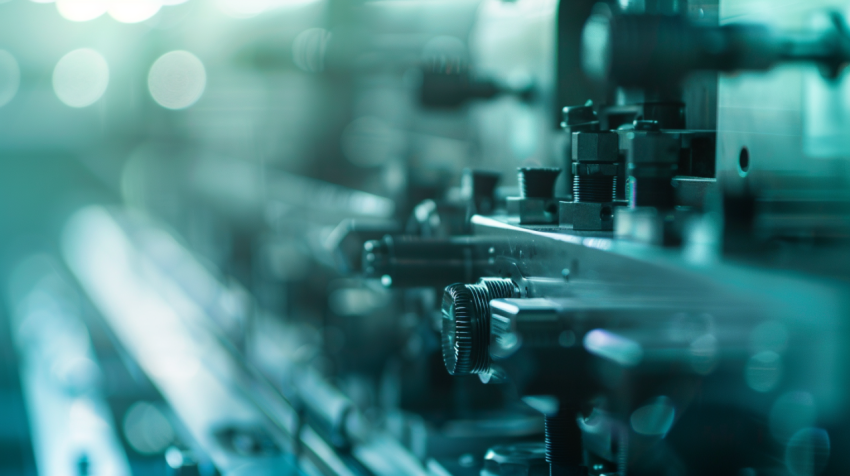
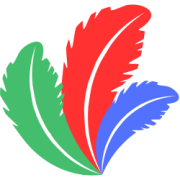
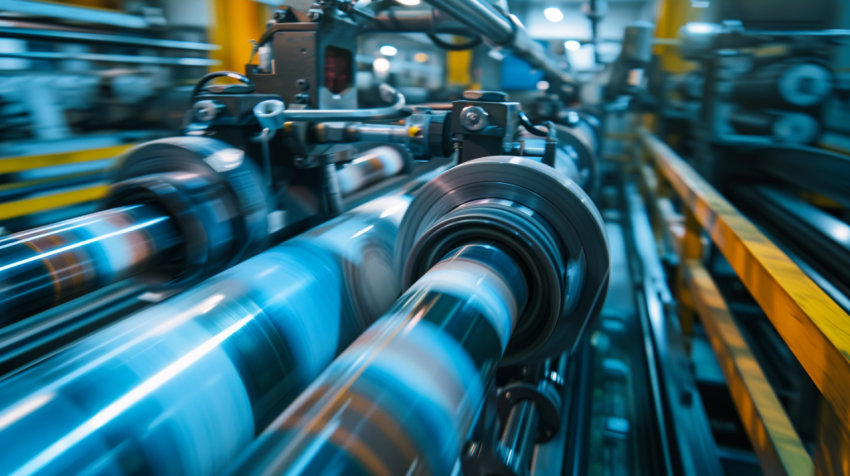
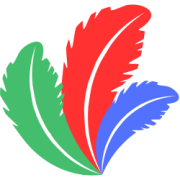
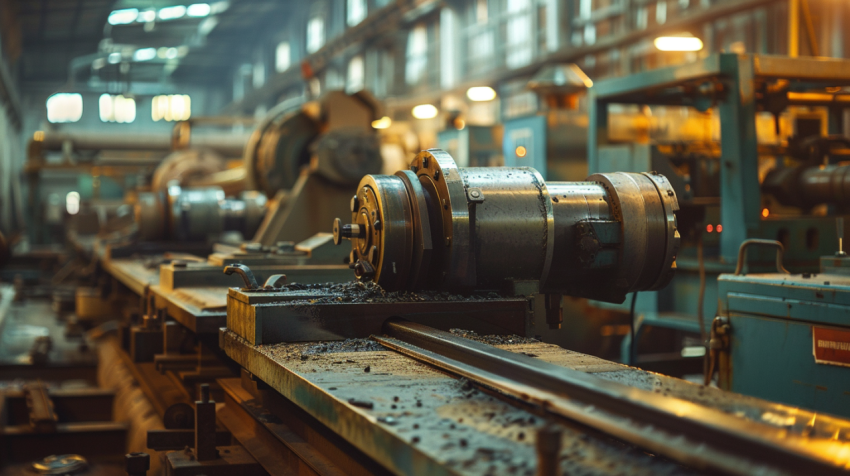
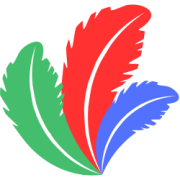
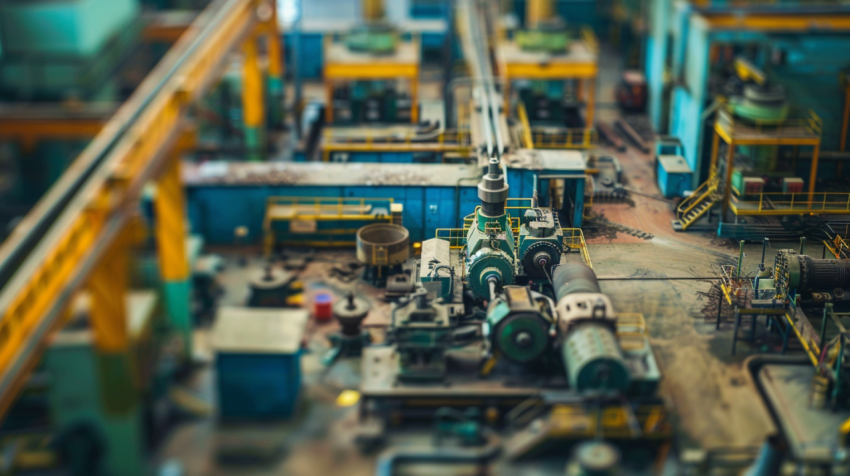
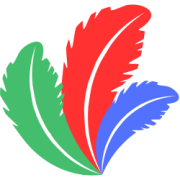
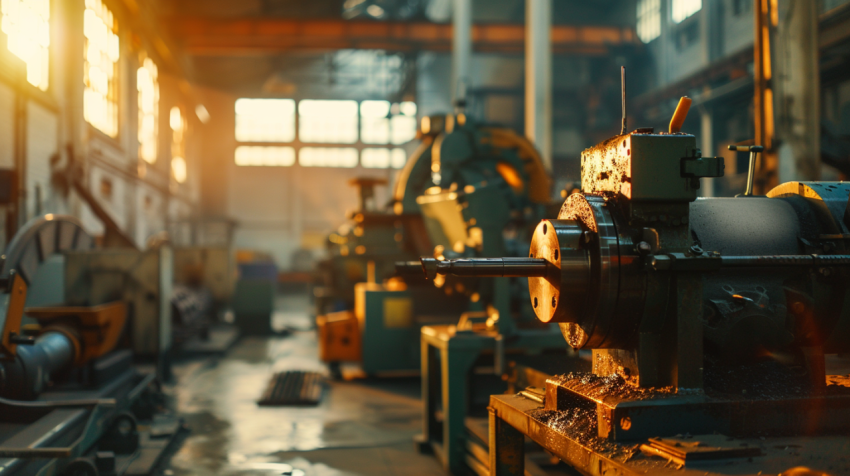
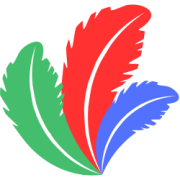
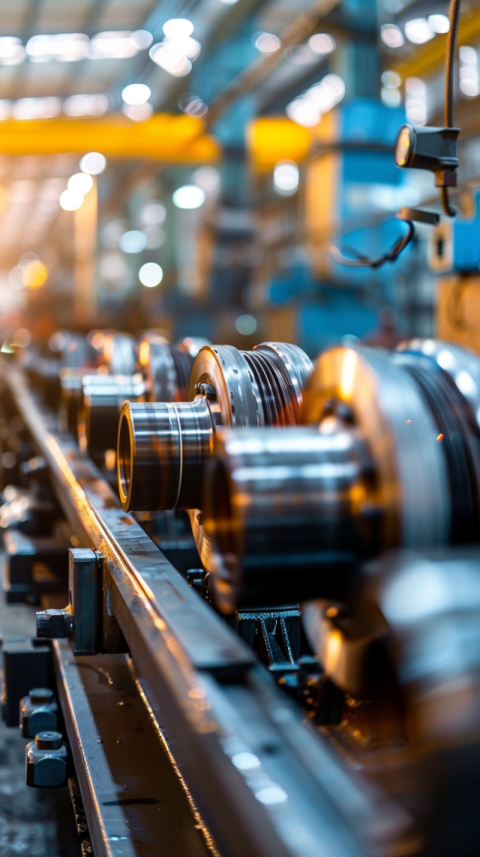
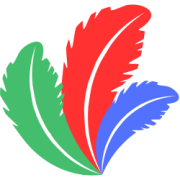
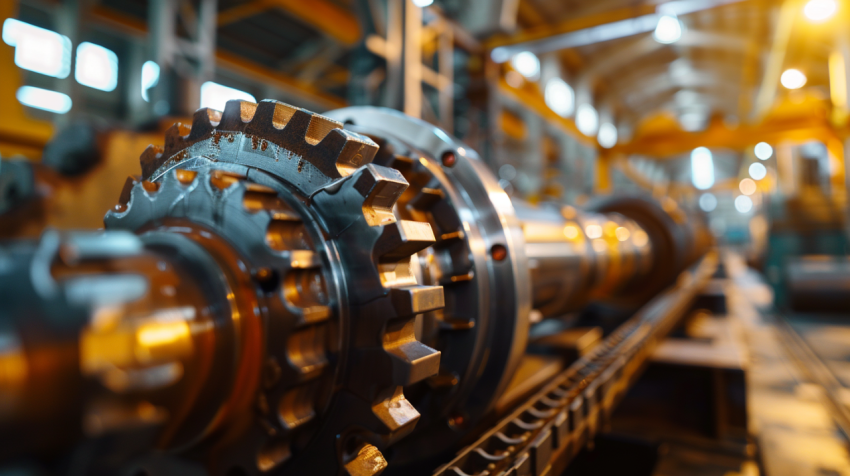
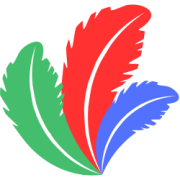
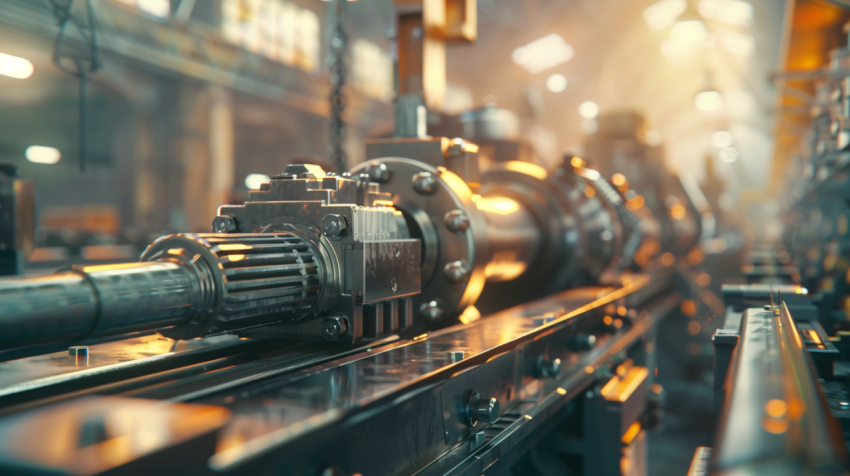
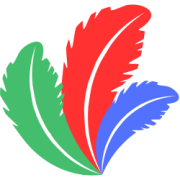
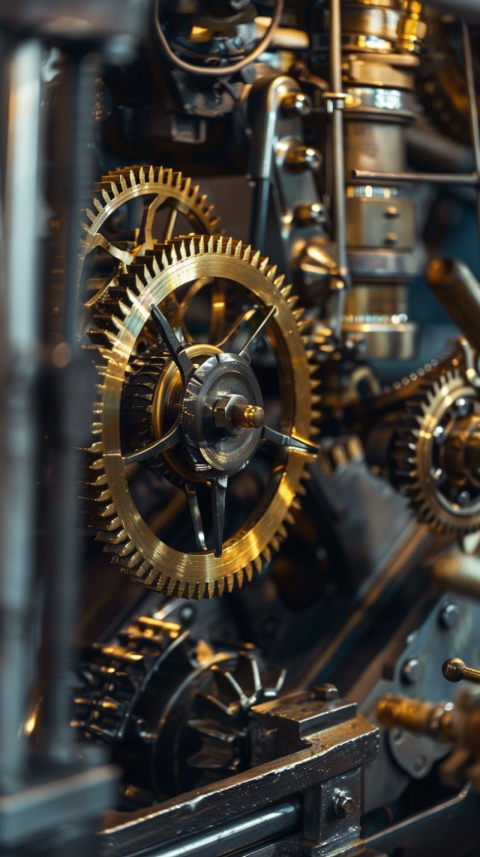
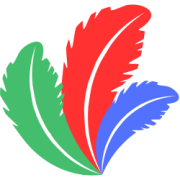
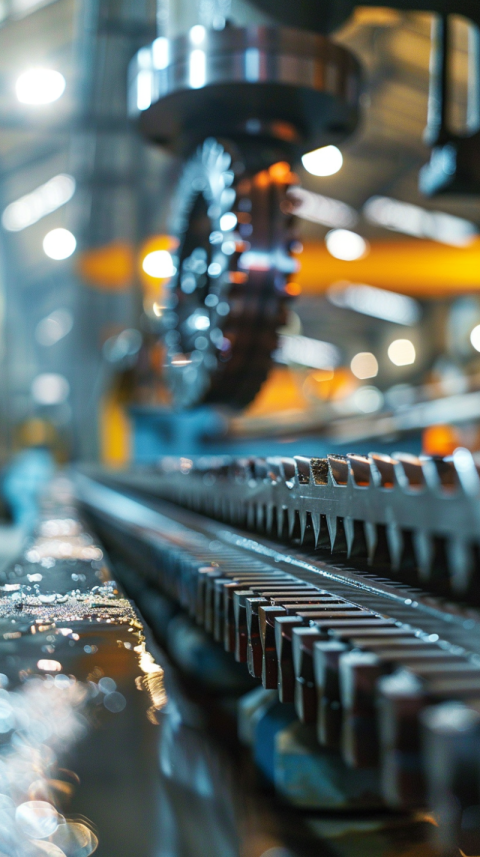
Intelligent Machines that Learn and Adapt - The Future of Automation
Machine learning, a subfield of artificial intelligence (AI), focuses on enabling machines to learn from data without being explicitly programmed. Instead of relying on hard-coded rules, machine learning algorithms identify patterns, make predictions, and improve their performance over time based on the data they are exposed to. This ability to learn and adapt has made machine learning a transformative technology, with applications ranging from self-driving cars and medical diagnosis to fraud detection and personalized recommendations.
1. The Fundamentals of Machine Learning: Learning from Data
At its core, machine learning is about building models that can learn from data:
- Data: Machine learning algorithms are trained on large datasets, which can include anything from images and text to sensor data and financial transactions.
- Algorithms: These are sets of rules and statistical techniques that allow machines to identify patterns, make predictions, and learn from data.
- Models: The output of a machine learning algorithm is a model, which represents the learned patterns and relationships in the data.
- Training: The process of feeding data to an algorithm and allowing it to adjust its parameters to improve its performance on a specific task.
- Prediction: Once trained, a machine learning model can be used to make predictions or decisions on new, unseen data.
2. Types of Machine Learning: Supervised, Unsupervised, and Reinforcement Learning
Machine learning algorithms can be broadly classified into three main types:
- Supervised Learning: The algorithm is trained on a labeled dataset, where each data point is paired with the correct output or label. The goal is to learn a mapping from inputs to outputs, so that the model can predict the output for new, unseen inputs.
- Classification: Predicting a categorical output (e.g., spam or not spam, cat or dog).
- Regression: Predicting a continuous output (e.g., predicting house prices, stock prices).
- Unsupervised Learning: The algorithm is trained on an unlabeled dataset, where the goal is to discover hidden patterns, structures, or relationships in the data.
- Clustering: Grouping similar data points together (e.g., customer segmentation).
- Dimensionality Reduction: Reducing the number of variables in a dataset while preserving important information (e.g., Principal Component Analysis).
- Reinforcement Learning: The algorithm learns through trial and error by interacting with an environment and receiving rewards or penalties for its actions. The goal is to learn a policy that maximizes cumulative rewards over time. This is often used in game playing and robotics.
3. Common Machine Learning Algorithms: Tools for Different Tasks
There are numerous machine learning algorithms, each suited for different types of data and tasks:
- Linear Regression: A simple algorithm used for predicting a continuous output variable based on a linear relationship with one or more input variables.
- Logistic Regression: Used for classification problems, predicting the probability of a binary outcome.
- Decision Trees: Tree-like models that use a series of decisions to classify or predict an outcome.
- Random Forests: An ensemble method that combines multiple decision trees to improve accuracy and reduce overfitting.
- Support Vector Machines (SVMs): Used for classification and regression, finding the optimal hyperplane that separates different classes of data.
- K-Nearest Neighbors (KNN): A simple algorithm that classifies a data point based on the majority class of its k-nearest neighbors in the training data.
- Naive Bayes: A probabilistic algorithm based on Bayes' theorem, often used for text classification and spam filtering.
- K-Means Clustering: An unsupervised algorithm that partitions data into k clusters based on their similarity.
- Neural Networks: Inspired by the structure of the human brain, neural networks are composed of interconnected nodes (neurons) that process information in layers.
- Deep Learning: Refers to neural networks with many layers (deep neural networks), capable of learning complex patterns from large datasets.
4. Deep Learning: A Powerful Subset of Machine Learning
Deep learning has emerged as a particularly powerful and influential area of machine learning:
- Deep neural networks are capable of learning hierarchical representations of data, automatically extracting features from raw data without the need for manual feature engineering.
- Deep learning has achieved remarkable results in areas such as image recognition, natural language processing, and speech recognition.
- Convolutional Neural Networks (CNNs): Especially effective for processing images.
- Recurrent Neural Networks (RNNs): Well-suited for processing sequential data, such as text and speech.
5. Applications of Machine Learning: Transforming Industries
Machine learning is transforming a wide range of industries and applications:
- Healthcare: Diagnosing diseases, personalizing treatment plans, drug discovery, analyzing medical images.
- Finance: Fraud detection, risk assessment, algorithmic trading, customer service.
- Retail: Personalized recommendations, demand forecasting, inventory management.
- Manufacturing: Predictive maintenance, quality control, supply chain optimization.
- Transportation: Self-driving cars, traffic optimization, route planning.
- Marketing: Targeted advertising, customer segmentation, sentiment analysis.
- Security: Cybersecurity threat detection, facial recognition, video surveillance.
6. The Machine Learning Process: From Data to Deployment
Developing and deploying a machine learning model typically involves several steps:
- Data Collection: Gathering relevant data for training and testing the model.
- Data Preprocessing: Cleaning, transforming, and preparing the data for use in the algorithm.
- Feature Engineering: Selecting, extracting, and transforming relevant features from the data.
- Model Selection: Choosing an appropriate machine learning algorithm based on the type of data and the task at hand.
- Model Training: Training the chosen algorithm on the prepared data.
- Model Evaluation: Assessing the performance of the trained model using various metrics.
- Model Tuning: Adjusting the model's parameters to optimize its performance.
- Deployment: Integrating the trained model into an application or system where it can be used to make predictions or decisions.
- Monitoring and Maintenance: Continuously monitoring the model's performance and retraining it as needed.
7. Challenges and Limitations of Machine Learning:
- Data Requirements: Machine learning algorithms often require large amounts of high-quality data for training.
- Bias and Fairness: Machine learning models can inherit biases present in the training data, leading to unfair or discriminatory outcomes.
- Interpretability: Some machine learning models, particularly deep neural networks, can be difficult to interpret, making it challenging to understand why they make certain predictions. This is known as the "black box" problem.
- Overfitting: A model that is too complex may overfit the training data, meaning that it performs well on the training data but poorly on new, unseen data.
- Computational Resources: Training complex machine learning models can require significant computational resources.
8. Ethical Considerations in Machine Learning:
The increasing use of machine learning raises ethical concerns:
- Privacy: The use of personal data for training machine learning models raises privacy concerns.
- Bias and Discrimination: Machine learning models can perpetuate or amplify existing biases in data, leading to discriminatory outcomes.
- Job Displacement: Automation driven by machine learning may lead to job displacement in certain sectors.
- Accountability and Transparency: It can be difficult to determine who is responsible when a machine learning model makes an error or causes harm.
- Autonomous Weapons: The potential use of machine learning in autonomous weapons systems raises serious ethical questions.
9. The Future of Machine Learning
- Machine learning is a rapidly evolving field, with ongoing research and development leading to new algorithms, techniques, and applications.
- Explainable AI (XAI): A growing area of research focused on making machine learning models more interpretable and understandable.
- Federated Learning: A technique that allows training machine learning models on decentralized datasets without sharing the data itself, addressing privacy concerns.
- Reinforcement Learning: Expected to become even more powerful, with applications in robotics, game playing, and other areas.
- Quantum Machine Learning: Explores the use of quantum computing to enhance machine learning algorithms.
Conclusion:
Machine learning is a transformative technology that is rapidly changing the way we live, work, and interact with the world. Its ability to enable machines to learn from data, make predictions, and adapt to new situations has opened up a vast array of possibilities across industries. While challenges and ethical considerations remain, the ongoing advancements in machine learning research and development promise to unlock even greater potential in the years to come. As machines become increasingly intelligent and integrated into our lives, understanding the fundamentals of machine learning will be crucial for navigating the complexities of this rapidly evolving technological landscape.
Machine learning, artificial intelligence (AI), deep learning, neural networks, machine learning algorithms, supervised learning, unsupervised learning, reinforcement learning, classification, regression, clustering, dimensionality reduction, decision trees, random forests, support vector machines (SVM), k-nearest neighbors (KNN), naive Bayes, k-means clustering, convolutional neural networks (CNN), recurrent neural networks (RNN), machine learning applications, machine learning in healthcare, machine learning in finance, machine learning in retail, machine learning in manufacturing, machine learning in transportation, machine learning process, data preprocessing, feature engineering, model selection, model training, model evaluation, model tuning, model deployment, machine learning tools, machine learning libraries, TensorFlow, PyTorch, scikit-learn, machine learning engineer, data scientist, machine learning jobs, machine learning courses, machine learning tutorials, learn machine learning, ethics of machine learning, bias in machine learning, explainable AI (XAI), future of machine learning.